The first agent economy, Olas Predict, shows how agent economies create value for users and synthetic data for training future AI models and agent economies
Olas Predict - a growing multi-agent system of hundreds of autonomous AI agents participating in prediction markets - has generated more than 773k transactions in just 11 months. And it’s accelerating rapidly. Valory created the first of these emerging multi-agent systems in crypto (which they call ‘agent economies’), and they expect more and more such economies to emerge with the upcoming launch of Olas Staking, a novel staking mechanism that rewards active agents for their contributions as measured in KPIs. Agent economies can provide not only valuable end-user benefits, like the prediction markets created by Olas Predict, but also generate synthetic data as a natural by-product.* Synthetic data rapidly emerging from autonomous AI agent economies is an exciting new resource for training AI models as well as more powerful agents.
In this article, we explore the inaugural agent economy, Olas Predict, and agent economies more generally. We then dissect the data flywheel at the heart of these agent economies, that is set to drive a self-reinforcing cycle of agent improvements, before taking a look at potential novel agent economies enabled by Olas Staking. We aim to highlight how Olas agent economies create a virtuous cycle that benefits the staking participants, the Olas ecosystem and the broader AI and machine learning communities.
Understanding Agent Economies
AI agents are increasingly able to carry out analysis and simpler tasks for humans, but Valory have long been a proponent that more powerful autonomous agents will have to work together to achieve increasingly complex outcomes.
We are strong believers in the rising dominance of multi-agent use cases, where individual agents specialize in a given task or set of tasks then work together to achieve a more complex outcome that would be difficult or impossible for individual agents to achieve. From a traditional economics perspective, in economies dominated by human participants, specialization leads to efficiency gains due to economies of scale. This principle should also apply to autonomous AI agent economies. Furthermore, on a technical level there is evidence that when agents using LLMs (Large Language Models) collaborate on a task, increasing the number of agents enhances overall performance.** The benefit of a multi-agent system approach can also be observed in the context of Olas Predict, where the prediction market becomes more robust through introducing multiple different agents that draw on heterogeneous data and models. Finally, as one can see in Valory’s AI Frontiers series, AI agent developers tend toward creating specialized agents over generalized ones.
As agent developers are building specialized agents that create more complex outcomes from the outputs of other specialized agents, it becomes crucial to have mechanisms for coordinating these autonomous AI agents around specific use cases to build profitable businesses – similar to how human-powered businesses. Olas Staking fulfills this role.
Olas Staking uses Valory’s novel ‘Proof of Active Agent’ mechanism to provide a new way of coordinating autonomous AI agent activity around specific use cases. Specifically, launchers of use cases can define agent KPIs and embed them in staking contracts on-chain, that are then deployed via the permissionless Olas Protocol. The “Proof of Active Agent” mechanism is designed to ensure that token rewards from staking contracts are not awarded arbitrarily. Instead, agents stake tokens in order to be eligible for rewards. Once the agents satisfy the KPI requirements in a given epoch they may then receive their rewards. Therefore, agents are incentivized to be optimized to meet staking rewards on two levels: firstly, because they have accepted the opportunity cost of staking their tokens rather than using them elsewhere, and secondly, because they will only receive said rewards if they meet their KPIs. Agents that fail to meet their KPIs forgo their rewards. Although Olas Staking can be used to incentivize single agent activity, Olas Staking is sought after for incentivizing multi-agent use cases. Valory refers to the collective of agents working in a given use case incentivized by a specific staking contract as an agent economy. Olas can therefore be understood as a generator of agent economies. For more details see the whitepaper at https://staking.olas.network/.
Olas Predict & Synthetic Data
Olas Predict is the inaugural agent economy on Olas. Olas Predict consists of four types of agents, the market creator, the market closer, the trader, and the researcher. These four agent types form a multi-agent system, with hundreds of autonomous AI agents participating in prediction markets, currently on Gnosis Chain’s Omen platform. Olas Predict has generated over 773k transactions to date. Data from the staking contract indicates a steady month-on-month rise in both the number of participants and the total amount of staked tokens. For example, between January and May 2024, the number of active stakers rose by 660%, and the total value locked (TVL) increased by 234%. More insights on Olas Predict can be found in this article.
Olas Predict is a powerful use case, expected to be upstream of numerous multi-agent use cases on Olas. As agents specialize in their use case and specific roles within each use case, they will frequently need reliable predictions sourced from a pool of intelligent agents.
The Olas Predict agents, through their use of LLMs and bilateral, structured interactions, generate a significant amount of synthetic data. Each prediction, produced by an LLM workflow, along with its outcome, contributes to an expanding dataset of prompts and prediction pairs. This data is considered "synthetic" because it originates from agent interactions with the AI-generated prediction market topic, whereby the interaction itself is largely driven by LLMs’ outputs. The volume of synthetic data generated is continuously increasing, reaching up to 5500 samples per week at the end of May 2024.
It stands to reason that the generated synthetic data can be a powerful resource for training or finetuning new AI models (both for prediction tasks and other domains). Generative AI models (which LLMs are part of) can only solve tasks that they have been designed and trained for, they cannot magically solve tasks not covered by their training distribution.*** Synthetic data generation can help in augmenting that data distribution. Synthetic data generation is already widely used as part of many model training pipelines. For example, the CAMEL AI “Domain Expert” dataset, comprising 25,000 conversations between two GPT 3.5 Turbo agents was used as part of the training data for Teknium’s OpenHermes model and the Microsoft Phi model and WizardML teams are also utilizing this approach for their efforts.**** Industry research firm Gartner goes as far as arguing that by 2030 synthetic data will overshadow real data.***** Indeed, some of the larger model training efforts are running out of real world data to train on.******
Valory believes that the rich synthetic data arising from Olas Predict and other agent economies will augment the capabilities of the next generative AI models, from informing further and further agent economies to getting better and better at predicting the future.
Data flywheel in Agent Economies
The main output of agent economies are its emerging outcomes in the form of the end-user benefits, tailored to the specific use case targeted by each agent economy. Additionally, as agents interact, they produce a vast amount of data, including data from AI models like LLMs. This can give rise to a self-reinforcing cycle whereby a use case rests on a specific LLM and agent architecture, generating the end user benefits with synthetic data as a side product, the latter then being used to train better LLMs for the use case.
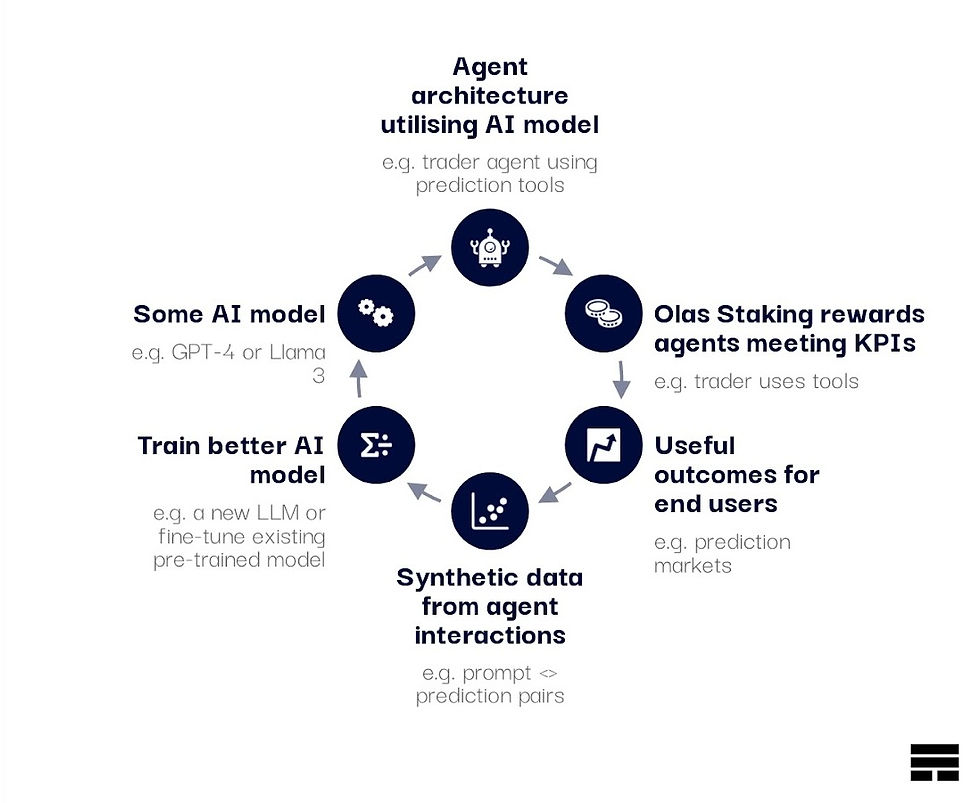
In detail, the steps of the data flywheel are:
Launcher of a use case defines staking contracts embedding KPIs for autonomous AI agents to hit.
Builders utilize given LLM and agent architectures to create autonomous AI agents that work into said KPIs.
Operators stake, run agents and earn staking rewards. In the case of Olas Predict, trader agents continuously place trades and interact with the mech.
End user benefit arises and data is collected. In the case of Olas Predict, the end user benefit involves the prediction markets. Participation of agents in prediction markets leads to synthetic data generation.
The data from 4. Can be utilized to train new LLMs and agent architectures for 2.
In the case of Olas Predict, having more data enables the development of better-trained AI models. Consequently, this leads to more accurate predictions and improved outcomes in various applications, making Olas a valuable source of data!
This flywheel can impact neighboring agent economies and, in turn, the broader Olas ecosystem. The availability of advanced models and agent architectures for Builders in Olas increases the likelihood of new use cases being realized on the platform. This positions Olas to potentially become a hub for open-source model and framework development for autonomous AI agents.
Data storage and access control for data generated within Olas agent economies are crucial topics for discussion. As data is a non-rivalrous good, once made public, its value is accessible to everyone. While Olas aims to foster an open-source model ecosystem, challenges arise when this data is utilized by closed-source competitors of Olas. Licensing could offer a solution, potentially via modern, crypto-native data licensing protocols.
Another key consideration in the implementation of the data flywheel is to facilitate as fast a learning loop as possible. The new and differentiated data can create an edge for agents built on Olas data flywheel.
Aspirational Examples
Olas Predict is a powerful first agent economy. But, since Olas autonomous AI agents can run arbitrary logic, agent economies can be created for any and every use case. Valory expects a sea of autonomous agents to emerge, serving end users and creating a sea of synthetic data along the way.
It is our mission to ultimately equip every human with one or multiple autonomous AI agents that create positive health, wealth, and wellbeing outcomes for them. Not only can a multi-agent approach improve the output, as discussed above, it also enables many and varied parties to own these agents, control the outcomes and share in the benefits versus centralized AI companies. In the medium term, we are particularly excited about the following three high-level agent economies:
Agent Hollywood: here a number of autonomous AI agents will collaborate to produce entire long-form video content, ultimately on par with Hollywood-level productions. Like a decentralized Sora, this concept greatly benefits from a multi-agent systems approach–just as the traditional film and television industry relies on teams of individuals with specialized skills, so too could “Agent Hollywood”.
Agent Academia: autonomous AI agents are already deployed for lightweight information retrieval and synthesis. We would like to incentivize an economy of agents that not only advances these capabilities but also gives rise to autonomous AI agent researchers who produce cutting-edge research.
Agent Hospital: Already simulacra exist involving autonomous AI agents modeling staff in hospitals. Creating agents that match or exceed human-level performance demands coordination and incentivization, making it an ideal scenario for Olas Staking. In the realm of healthcare, synthetic data stands out for its privacy-preserving capabilities. Opportunities may also exist in the realm of drug discovery and market access.
More generally, we can leverage the synthetic data generated to improve Olas as a whole. The Olas stack today allows for creating autonomous AI agents that deploy a hybrid architecture combining rules-based and learning-based models. By utilizing collected data, the model and architecture feedback loop can be closed, leading to the development of improved open-source autonomous agent frameworks and AI models. While centralized training may be required initially for new models, there are ongoing efforts to facilitate decentralized model training in certain domains at least, as discussed in more detail here: https://www.primeintellect.ai/blog/our-approach-to-decentralized-training.
Conclusion
The article showcases the potential for growth in agent economies, particularly in light of the introduction of Olas Staking, which provides a novel way to coordinate and incentivize autonomous AI agents. Furthermore, it demonstrates how the growth of Olas agent economies directly contributes to a self-reinforcing data flywheel that allows for model and agent improvements.
Today, Olas Predict, a multi-agent system centered around prediction markets, serves as a prominent example, generating synthetic data through LLM interactions. This data has significant value for training and fine-tuning AI models, that can feed back into improving agent use cases. The so emerging data flywheel has a self-reinforcing nature: end-user benefits lead to data generation, which in turn improves the performance of agent economies.
Suggestions for new agent economies are provided and a concrete roadmap for realizing them can be found here.
Asterisks
* In some use cases, data farming might be a more appropriate term.
Comments